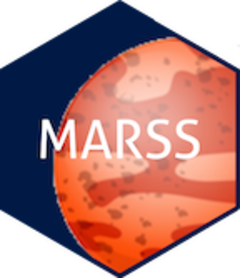
MARSS Contemporaneous Residuals
MARSSresiduals_ttt.Rd
Calculates the standardized (or auxiliary) contemporaneous residuals, aka the residuals and their variance conditioned on the data up to time MARSSresiduals(object, type="tt")
.
Arguments
- object
An object of class
marssMLE
.- method
Algorithm to use. Currently only "SS".
- normalize
TRUE/FALSE See details.
- silent
If TRUE, don't print inversion warnings.
- fun.kf
Can be ignored. This will change the Kalman filter/smoother function from the value in object$fun.kf if desired.
Value
A list with the following components
- model.residuals
The observed contemporaneous model residuals: data minus the model predictions conditioned on the data 1 to t. A n x T matrix. NAs will appear where the data are missing.
- state.residuals
All NA. There are no contemporaneous residuals for the states.
- residuals
The residuals.
model.residuals
are in rows 1:n andstate.residuals
are in rows n+1:n+m.- var.residuals
The joint variance of the residuals conditioned on observed data from 1 to t-. This only has values in the 1:n,1:n upper block for the model residuals.
- std.residuals
The Cholesky standardized residuals as a n+m x T matrix. This is
residuals
multiplied by the inverse of the lower triangle of the Cholesky decomposition ofvar.residuals
. The model standardized residuals associated with the missing data are replaced with NA. Note because the contemporaneous state residuals do not exist, rows n+1:n+m are all NA.- mar.residuals
The marginal standardized residuals as a n+m x T matrix. This is
residuals
multiplied by the inverse of the diagonal matrix formed by the square-root of the diagonal ofvar.residuals
. The model marginal residuals associated with the missing data are replaced with NA.- bchol.residuals
Because state residuals do not exist, this will be equivalent to the Cholesky standardized residuals,
std.residuals
.- E.obs.residuals
The expected value of the model residuals conditioned on the observed data 1 to t. Returned as a n x T matrix.
- var.obs.residuals
The variance of the model residuals conditioned on the observed data. Returned as a n x n x T matrix. For observed data, this will be 0. See
MARSSresiduals.tT()
for a discussion of these residuals and where they might be used.- msg
Any warning messages. This will be printed unless Object$control$trace = -1 (suppress all error messages).
Details
This function returns the conditional expected value (mean) and variance of the model contemporaneous residuals. 'conditional' means in this context, conditioned on the observed data up to time
Model residuals
MARSSresiduals.tt
fits the model using the data up to time residuals
.
var.residuals
returned by the function is the conditional variance of the residuals conditioned on the data up to MARSSkfss
in Vtt
.
Standardized residuals
std.residuals
are Cholesky standardized residuals. These are the residuals multiplied by the inverse of the lower triangle of the Cholesky decomposition of the variance matrix of the residuals:
The interpretation of the Cholesky standardized residuals is not straight-forward when the
mar.residuals
are the marginal standardized residuals. These are the residuals multiplied by the inverse of the diagonal matrix formed from the square-root of the diagonal of the variance matrix of the residuals:
diag(diag(A))
. These residuals will be correlated if the variance matrix is non-diagonal.
Normalized residuals
If normalize=FALSE
, the unconditional variance of
MARSSresiduals()
returns the residuals defined as in the first equations. To get normalized residuals (second equation) as used in Harvey et al. (1998), then use normalize=TRUE
. In that case the unconditional variance of residuals will be
References
Holmes, E. E. 2014. Computation of standardized residuals for (MARSS) models. Technical Report. arXiv:1411.0045.
Examples
dat <- t(harborSeal)
dat <- dat[c(2,11),]
fit <- MARSS(dat)
#> Success! abstol and log-log tests passed at 26 iterations.
#> Alert: conv.test.slope.tol is 0.5.
#> Test with smaller values (<0.1) to ensure convergence.
#>
#> MARSS fit is
#> Estimation method: kem
#> Convergence test: conv.test.slope.tol = 0.5, abstol = 0.001
#> Estimation converged in 26 iterations.
#> Log-likelihood: 11.74016
#> AIC: -9.480311 AICc: -6.3692
#>
#> Estimate
#> R.diag 0.0115
#> U.X.CoastalEstuaries 0.0613
#> U.X.OR.NorthCoast 0.0510
#> Q.(X.CoastalEstuaries,X.CoastalEstuaries) 0.0147
#> Q.(X.OR.NorthCoast,X.OR.NorthCoast) 0.0122
#> x0.X.CoastalEstuaries 7.3823
#> x0.X.OR.NorthCoast 6.2707
#> Initial states (x0) defined at t=0
#>
#> Standard errors have not been calculated.
#> Use MARSSparamCIs to compute CIs and bias estimates.
#>
# Returns a matrix
MARSSresiduals(fit, type="tt")$std.residuals
#> [,1] [,2] [,3] [,4] [,5] [,6]
#> CoastalEstuaries -0.05418676 -0.2062863 0.566365934 1.005768 NA 0.2204673
#> OR.NorthCoast NA NA -0.001882139 NA NA NA
#> X.CoastalEstuaries NA NA NA NA NA NA
#> X.OR.NorthCoast NA NA NA NA NA NA
#> [,7] [,8] [,9] [,10] [,11] [,12]
#> CoastalEstuaries 2.040538 1.2425972 -0.798201 -0.8746660 1.313388 -1.1010233
#> OR.NorthCoast NA -0.1395806 1.155472 0.1779561 0.343844 -0.8332411
#> X.CoastalEstuaries NA NA NA NA NA NA
#> X.OR.NorthCoast NA NA NA NA NA NA
#> [,13] [,14] [,15] [,16] [,17]
#> CoastalEstuaries 1.784981 -0.3351368 -0.7114291 NA 0.5726369
#> OR.NorthCoast 1.616618 0.6909545 1.0951714 0.03597429 -0.9304752
#> X.CoastalEstuaries NA NA NA NA NA
#> X.OR.NorthCoast NA NA NA NA NA
#> [,18] [,19] [,20] [,21] [,22]
#> CoastalEstuaries -0.7677528 -0.31659312 -2.3132481 -0.4943444 0.05447392
#> OR.NorthCoast 1.6416251 -0.08266883 -0.6865966 -1.3199941 -0.07484734
#> X.CoastalEstuaries NA NA NA NA NA
#> X.OR.NorthCoast NA NA NA NA NA
#> [,23] [,24] [,25] [,26] [,27]
#> CoastalEstuaries 0.01643296 NA -0.8768965 NA NA
#> OR.NorthCoast NA 0.2166126 -2.1429904 -1.520447 -0.8263151
#> X.CoastalEstuaries NA NA NA NA NA
#> X.OR.NorthCoast NA NA NA NA NA
#> [,28] [,29] [,30]
#> CoastalEstuaries NA NA NA
#> OR.NorthCoast 0.9151561 0.7476442 NA
#> X.CoastalEstuaries NA NA NA
#> X.OR.NorthCoast NA NA NA
# Returns a data frame in long form
residuals(fit, type="tt")
#> type .rownames name t value .fitted .resids .sigma
#> 1 ytt CoastalEstuaries model 1 7.434848 7.438695 -3.847067e-03 0.07099643
#> 2 ytt CoastalEstuaries model 2 7.462789 7.475908 -1.311903e-02 0.06359622
#> 3 ytt CoastalEstuaries model 3 7.641084 7.605603 3.548099e-02 0.06264676
#> 4 ytt CoastalEstuaries model 4 7.851661 7.788765 6.289604e-02 0.06253534
#> 5 ytt CoastalEstuaries model 5 NA 7.850112 NA NA
#> 6 ytt CoastalEstuaries model 6 7.959975 7.948468 1.150614e-02 0.05218980
#> 7 ytt CoastalEstuaries model 7 8.391176 8.265783 1.253930e-01 0.06145096
#> 8 ytt CoastalEstuaries model 8 8.555837 8.478301 7.753586e-02 0.06239822
#> 9 ytt CoastalEstuaries model 9 8.392990 8.442882 -4.989279e-02 0.06250655
#> 10 ytt CoastalEstuaries model 10 8.343554 8.398237 -5.468331e-02 0.06251908
#> 11 ytt CoastalEstuaries model 11 8.700847 8.618733 8.211373e-02 0.06252053
#> 12 ytt CoastalEstuaries model 12 8.477828 8.546665 -6.883674e-02 0.06252070
#> 13 ytt CoastalEstuaries model 13 8.935904 8.824305 1.115983e-01 0.06252072
#> 14 ytt CoastalEstuaries model 14 8.824089 8.845042 -2.095299e-02 0.06252072
#> 15 ytt CoastalEstuaries model 15 8.775704 8.820183 -4.447906e-02 0.06252072
#> 16 ytt CoastalEstuaries model 16 NA 8.881530 NA NA
#> 17 ytt CoastalEstuaries model 17 9.068892 9.039007 2.988524e-02 0.05218881
#> 18 ytt CoastalEstuaries model 18 8.956866 9.004046 -4.717908e-02 0.06145087
#> 19 ytt CoastalEstuaries model 19 9.007122 9.026877 -1.975484e-02 0.06239821
#> 20 ytt CoastalEstuaries model 20 8.663196 8.807790 -1.445932e-01 0.06250655
#> 21 ytt CoastalEstuaries model 21 8.778326 8.809232 -3.090596e-02 0.06251908
#> 22 ytt CoastalEstuaries model 22 8.880586 8.877180 3.405739e-03 0.06252053
#> 23 ytt CoastalEstuaries model 23 8.941545 8.940518 1.027400e-03 0.06252070
#> 24 ytt CoastalEstuaries model 24 NA 9.001865 NA NA
#> 25 ytt CoastalEstuaries model 25 8.870242 8.916006 -4.576419e-02 0.05218881
#> 26 ytt CoastalEstuaries model 26 NA 8.977353 NA NA
#> 27 ytt CoastalEstuaries model 27 NA 9.038700 NA NA
#> 28 ytt CoastalEstuaries model 28 NA 9.100047 NA NA
#> 29 ytt CoastalEstuaries model 29 NA 9.161394 NA NA
#> 30 ytt CoastalEstuaries model 30 NA 9.222741 NA NA
#> 31 ytt OR.NorthCoast model 1 NA 6.321668 NA NA
#> 32 ytt OR.NorthCoast model 2 NA 6.372664 NA NA
#> 33 ytt OR.NorthCoast model 3 6.423247 6.423346 -9.863442e-05 0.05240548
#> 34 ytt OR.NorthCoast model 4 NA 6.474341 NA NA
#> 35 ytt OR.NorthCoast model 5 NA 6.525337 NA NA
#> 36 ytt OR.NorthCoast model 6 NA 6.576333 NA NA
#> 37 ytt OR.NorthCoast model 7 NA 6.627329 NA NA
#> 38 ytt OR.NorthCoast model 8 6.638568 6.644196 -5.628105e-03 0.04032153
#> 39 ytt OR.NorthCoast model 9 6.906755 6.834278 7.247679e-02 0.06272484
#> 40 ytt OR.NorthCoast model 10 6.916715 6.905147 1.156766e-02 0.06500290
#> 41 ytt OR.NorthCoast model 11 7.016610 6.994154 2.245576e-02 0.06530798
#> 42 ytt OR.NorthCoast model 12 6.898715 6.953167 -5.445231e-02 0.06535000
#> 43 ytt OR.NorthCoast model 13 7.288244 7.182589 1.056554e-01 0.06535581
#> 44 ytt OR.NorthCoast model 14 7.355002 7.309843 4.515845e-02 0.06535662
#> 45 ytt OR.NorthCoast model 15 7.553287 7.481710 7.157682e-02 0.06535673
#> 46 ytt OR.NorthCoast model 16 7.539027 7.536676 2.351163e-03 0.06535674
#> 47 ytt OR.NorthCoast model 17 7.424165 7.484978 -6.081283e-02 0.06535675
#> 48 ytt OR.NorthCoast model 18 7.824446 7.717155 1.072913e-01 0.06535675
#> 49 ytt OR.NorthCoast model 19 7.753624 7.759027 -5.402966e-03 0.06535675
#> 50 ytt OR.NorthCoast model 20 7.689371 7.734245 -4.487372e-02 0.06535675
#> 51 ytt OR.NorthCoast model 21 7.553287 7.639557 -8.627052e-02 0.06535675
#> 52 ytt OR.NorthCoast model 22 7.677400 7.682292 -4.891778e-03 0.06535675
#> 53 ytt OR.NorthCoast model 23 NA 7.733288 NA NA
#> 54 ytt OR.NorthCoast model 24 7.829233 7.817244 1.198862e-02 0.05534590
#> 55 ytt OR.NorthCoast model 25 7.484369 7.621765 -1.373964e-01 0.06411433
#> 56 ytt OR.NorthCoast model 26 7.404888 7.504001 -9.911363e-02 0.06518718
#> 57 ytt OR.NorthCoast model 27 7.409742 7.463728 -5.398592e-02 0.06533333
#> 58 ytt OR.NorthCoast model 28 7.675546 7.615737 5.980866e-02 0.06535351
#> 59 ytt OR.NorthCoast model 29 7.798113 7.749249 4.886326e-02 0.06535630
#> 60 ytt OR.NorthCoast model 30 NA 7.800245 NA NA
#> .std.resids
#> 1 -0.054186758
#> 2 -0.206286276
#> 3 0.566365934
#> 4 1.005767860
#> 5 NA
#> 6 0.220467252
#> 7 2.040537901
#> 8 1.242597244
#> 9 -0.798201023
#> 10 -0.874666007
#> 11 1.313388330
#> 12 -1.101023270
#> 13 1.784980949
#> 14 -0.335136785
#> 15 -0.711429056
#> 16 NA
#> 17 0.572636946
#> 18 -0.767752824
#> 19 -0.316593118
#> 20 -2.313248083
#> 21 -0.494344408
#> 22 0.054473923
#> 23 0.016432959
#> 24 NA
#> 25 -0.876896499
#> 26 NA
#> 27 NA
#> 28 NA
#> 29 NA
#> 30 NA
#> 31 NA
#> 32 NA
#> 33 -0.001882139
#> 34 NA
#> 35 NA
#> 36 NA
#> 37 NA
#> 38 -0.139580626
#> 39 1.155471842
#> 40 0.177956078
#> 41 0.343844019
#> 42 -0.833241083
#> 43 1.616618091
#> 44 0.690954477
#> 45 1.095171415
#> 46 0.035974293
#> 47 -0.930475250
#> 48 1.641625053
#> 49 -0.082668833
#> 50 -0.686596598
#> 51 -1.319994101
#> 52 -0.074847339
#> 53 NA
#> 54 0.216612615
#> 55 -2.142990433
#> 56 -1.520446816
#> 57 -0.826315058
#> 58 0.915156126
#> 59 0.747644217
#> 60 NA